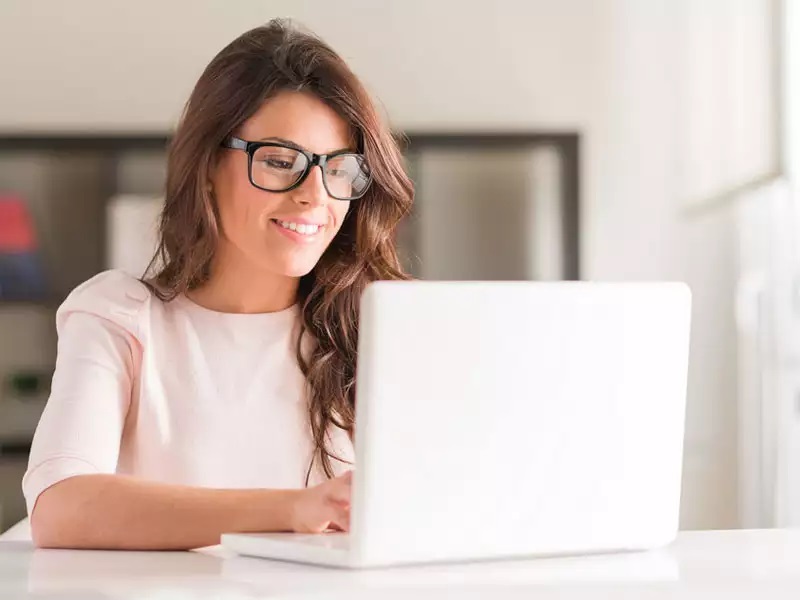
As data science evolves, new tools and methods reshape the industry, with synthetic data emerging as a powerful resource. For those considering a data science course in Mumbai, understanding synthetic data is critical and empowering. Synthetic data enables machine learning algorithms to train on artificially manufactured datasets that mimic real-world data without the complexities of privacy concerns or limited data availability. Let’s discuss the growing trend of synthetic data in machine learning, its advantages, typical applications, challenges, and why it’s revolutionizing how machine learning solutions are developed.
What is Synthetic Data?
Synthetic data refers to data generated by algorithms to resemble actual data without being directly sourced from real-life examples. This data can be created through various techniques, such as simulations or generative models, to capture essential patterns and structures within a dataset. It is especially useful in machine learning, where extensive, high-quality datasets are essential for training models effectively. With synthetic data, developers can bypass some of the limitations of traditional data collection, enabling more extensive experimentation and model accuracy.
Why Synthetic Data is Gaining Popularity
The rapid rise in synthetic data use across industries is due to several critical factors that address specific challenges in data science and machine learning. Synthetic data is not just a theoretical concept; it’s a practical solution to real-world challenges.
- Privacy and Security: Real-world datasets, especially those involving sensitive information like healthcare or financial records, are tightly regulated for privacy reasons. Synthetic data offers an alternative that maintains the patterns and characteristics of real data without risking exposure to actual user information.
- Data Availability and Cost: Real datasets can be challenging to collect, particularly in fields requiring extensive testing or complex data relationships. Generating synthetic data is faster, less expensive, and can be done on demand, making it easier for organizations to create tailored datasets suited to their models.
- Balanced and Comprehensive Datasets: Real datasets are often biased or lack sufficient examples for specific categories. Synthetic data enables balanced representation, supporting robust machine-learning models across varied inputs. For instance, synthetic data can simulate rare conditions in fields like medical diagnosis, creating a dataset with better coverage.
- Enhanced Testing and Experimentation: Because synthetic data is generated as needed, it provides flexibility for testing under various hypothetical conditions, such as extreme weather events or market shifts. It’s invaluable for researchers and businesses aiming to see how models perform in diverse scenarios without waiting for those scenarios to occur.
These advantages explain why professionals, particularly those exploring a data science course in Mumbai, are keenly interested in synthetic data’s potential to transform machine learning applications.
Applications of Synthetic Data in Machine Learning
Synthetic data impacts multiple fields, with applications becoming more sophisticated and varied. Here are some areas where synthetic data is already proving to be invaluable:
- Healthcare: Synthetic data allows for the creation of large datasets representing patient conditions without disclosing personal health information. It’s not just about data; it’s about accelerating innovation and improving patient care. Thanks to synthetic data, researchers can study trends, improve diagnostic tools, and explore drug efficacy using anonymized yet realistic datasets.
- Autonomous Vehicles: Autonomous technology requires vast training datasets containing innumerable driving scenarios. However, capturing real-world data for every possible situation is impractical. Synthetic data, including simulated pedestrians, vehicles, and road conditions, makes autonomous systems safer and more reliable.
- Finance and Insurance: Financial modeling relies on patterns that synthetic data can replicate, which is especially useful for creating datasets without disclosing personal or proprietary financial information. Insurers use synthetic data to test risk models, simulate fraud, and predict market behavior under hypothetical circumstances.
- Retail and Customer Behavior Analysis: Companies can use synthetic data to simulate customer interactions, purchasing behaviors, and seasonal trends. It enables them to train recommendation engines, predict inventory needs, and refine marketing strategies without requiring exhaustive data from actual customers.
These applications show that synthetic data doesn’t just supplement existing data sources; it provides new flexibility and innovation, especially relevant for data science practitioners.
How Synthetic Data is Generated
Several methods exist for generating synthetic data, each with its strengths and practical use cases. These methods are efficient and cost-effective, making synthetic data generation accessible to a wide range of applications.
- Random Sampling: Simple methods, like random sampling, create synthetic datasets by randomly selecting data from existing distributions. While essential, it’s a practical approach for small-scale datasets with limited complexity.
- Simulations: Simulation-based synthetic data involves creating hypothetical scenarios using mathematical models. For example, a retail company might simulate holiday shopping traffic, providing data that reflects a high-demand season without waiting for the actual period.
- Generative Models (GANs and VAEs): Generative Adversarial Networks (GANs) and Variational Autoencoders (VAEs) are machine learning models specifically designed to generate synthetic data by learning from real datasets. GANs are particularly popular as they allow for the creation of highly realistic synthetic data, often indistinguishable from real data.
- Data Augmentation: In some cases, synthetic data can be generated by modifying real data. For instance, images can be cropped, rotated, or recolored to create new variations that increase dataset diversity. This strategy is frequently employed in computer vision applications that require visual data from several angles.
By leveraging these techniques, synthetic data offers unique solutions that would be difficult or costly to achieve with real-world data alone.
Challenges and Limitations of Synthetic Data
While synthetic data offers many advantages, it also has challenges that need careful consideration:
- Quality and Realism: Not all synthetic data is equally realistic, and models trained on low-quality data may not generalize well when applied to real-world situations. Therefore, it’s crucial to ensure high-quality generation techniques, such as advanced GANs, are used to add value to synthetic data.
- Bias and Variability: Although synthetic data can correct biases in real datasets, it can also introduce new biases if the data generation process is not well-controlled. Addressing these biases requires a careful approach, particularly when generating data for sensitive applications like healthcare or law enforcement.
- Technical Complexity: Generating high-quality synthetic data often requires advanced data science and machine learning expertise, adding to the resource requirements for effective implementation. That is where a data science course in Mumbai or similar training programs can play an essential role, as they provide the necessary skills for creating and managing synthetic datasets.
- Legal and Ethical Concerns: Although synthetic data bypasses some regulatory hurdles, it is still subject to ethical considerations, primarily when representing sensitive domains. Organizations must ensure that their use of synthetic data aligns with ethical standards and regulatory expectations.
These challenges highlight that synthetic data is promising but not a universal solution. Its implementation must be balanced with attention to quality, control, and ethics.
Conclusion
Synthetic data transforms how machine learning models are developed, tested, and refined. It provides a flexible, scalable alternative to real-world data that addresses many industry challenges, from privacy to availability and cost. For aspiring data scientists and professionals, particularly those considering a data science course in Mumbai, mastering synthetic data techniques offers a valuable skill set that aligns with current industry needs and future trends. As synthetic data technology advances, its potential applications across industries continue to grow, making it a key trend for anyone interested in machine learning and data science.
Business Name: Data Science, Data Analyst and Business Analyst Course in Mumbai
Address: 1304, 13th floor, A wing, Dev Corpora, Cadbury junction, Eastern Express Highway, Thane, Mumbai, Maharashtra 400601 Phone: 095132 58922